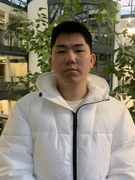
Dr. Jialong Li
- Address
- Max-Planck-Institut für Informatik
Saarland Informatics Campus
Campus E1 4
66123 Saarbrücken - Location
- E1 4 - 518
- Phone
- +49 681 9325 0
- Fax
- +49 681 9325 5719
- Get email via email
I am a postdoc researcher at Max Planck Institute for Informatics (MPF-INF). My research interests include optical networks, optical communications, and computer networks. Before joining MPI-INF, I received my B.E. and Ph.D. degree in Electronic Engineering from Tsinghua University in 2016 and 2021, respectively.
For more information, please see my personal website: https://franklee94.github.io/.
Conferences
Reviewer, The 11th International Conference on Wireless Communications and Signal Processing (WCSP 2019)
Journals
Reviewer, IEEE Communications Letters
Reviewer, IEEE/OSA Journal of Optical Communications and Networking
At MPI / Saarland University:
At Tsinghua University:
November 2021 - now:
Postdoctoral Researcher at Max Planck Institute for Informatics
August 2016 - October 2021:
Ph.D. in Electronic Engineering, Tsinghua University
August 2012 - July 2016:
B.S. in Electronic Engineering, Tsinghua University