Markerless Reconstruction of Dynamic Scenes
Markerless Reconstruction of Dynamic Scenes
Reconstruction of detailed animation models from multi-video data
The development of new algorithms to reconstruct geometry and appearance models of dynamic scenes in the real world is an important goal of graphics and vision research. Captured dynamic models of humans and more general scenes are very important for computer animation and visual effects. Reconstruction of the dynamic environment with sensors is also highly important for autonomous robots and vehicles. It is also the precondition for 3D video and virtual replay production in TV broadcasting. Reconstruction of human motion, in particular, is of increasing importance in biomechanics, sports sciences, and humancomputer interaction. The development of new sensors and new see-through displays also brings us closer to truly believable augmented reality. The precondition for this is also having algorithms to measure models of the real world in real-time.
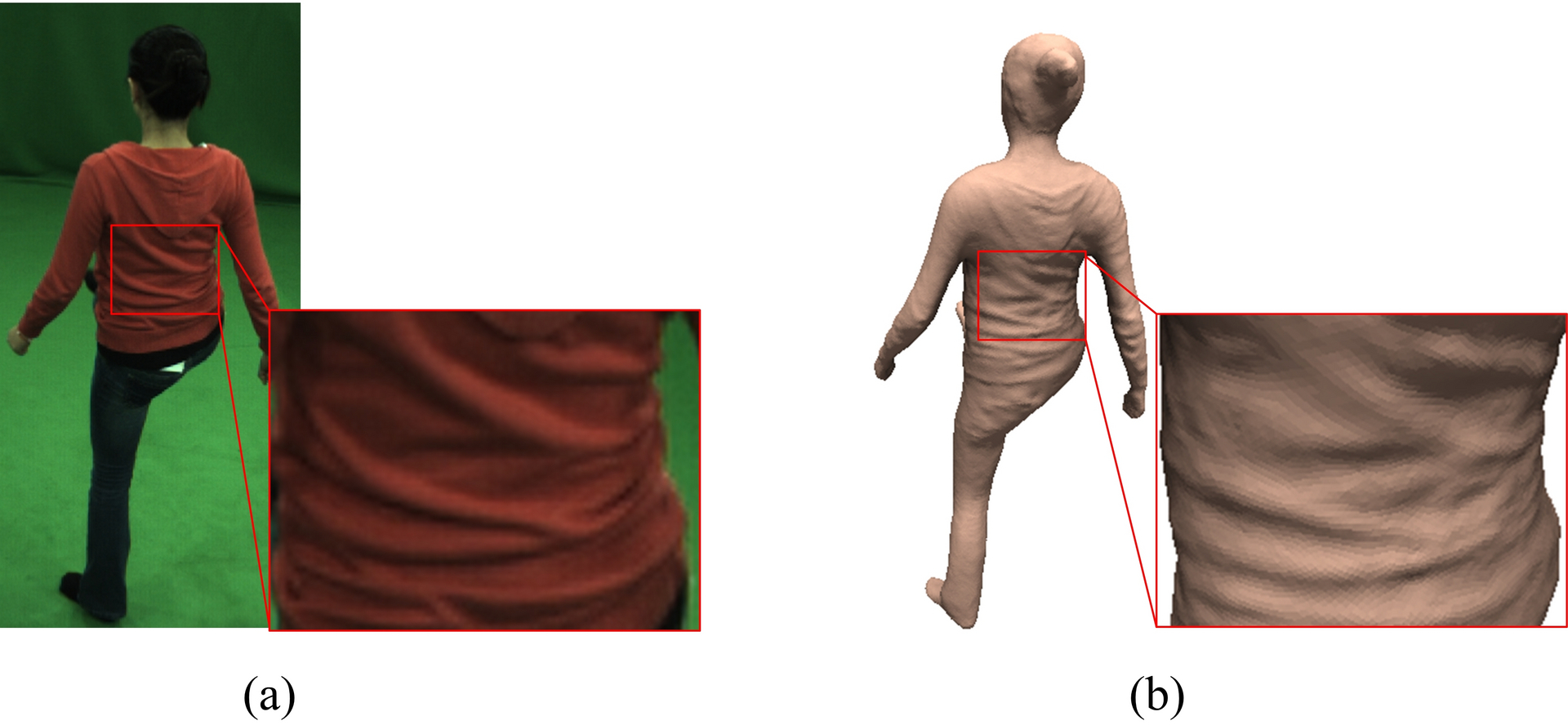
Figure 1: Performance capture with a stereo camera
The goal of our research is thus the development of new performance capture algorithms. These algorithms reconstruct detailed models of geometry, motion, and appearance of dynamic scenes from multi-view video data. The reconstruction is to be performed without optical interference with the scene, for instance, fiducial markers, as are commonly used in motion capture. In the past, we have pioneered the development of performance capture algorithms that are able to reconstruct detailed models of persons in general apparel or of arbitrary moving objects from multi-view video. Unfortunately, the application of these methods is constrained to indoor studios with many cameras, controlled lighting, and controlled backgrounds. In the reporting period. we therefore began to redefine the algorithmic foundations of dynamic scene reconstruction to enable, in the long run, performance capture of arbitrarily complex outdoor scenes under uncontrolled lighting and with only a few cameras.
In the reporting period, we developed some of the first methods for the detailed marker-less skeletal motion capture of humans and animals in outdoor scenes with few cameras (2 – 3). Another milestone was the development of new inverse rendering algorithms that allow us to measure models of appearance, illumination, and detailed geometry from video footage recorded under uncontrolled conditions. This new methodology is the basis for other important results: the first approach to capture relightable 3D videos in uncontrolled scenes, the first methods to capture highly detailed dynamic face models from stereo or monocular video, and the first approach for detailed full body performance capture with a single stereo camera [ see figure 1 ]. An important new strand of research is also the development of algorithms for marker-less hand motion capture from a few cameras, or even from a single camera view [ see figure 2 ].
Reconstruction of static and dynamic scenes with depth sensors
New types of depth cameras measure 2.5 D scene geometry in real-time. These new sensors are cheap and can be an important tool to bring reconstruction methods for static and dynamic scenes to a broader user community. Unfortunately, depth camera data is very noisy, has low resolution, and exhibits a systematic measurement bias. We have, therefore, developed methods to calibrate depth sensors, eliminate noise, and algorithmically increase the camera resolution. Furthermore, we are working on new algorithms to capture geometry models of even large scenes with such sensors.
Additionally, we research new methods to capture human skeletal motion and body shape in real-time from a single depth camera. In this context, we have also developed some of the first approaches for real-time capture of general deformable shapes with a single camera.
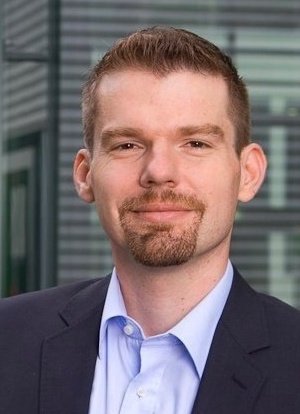
About the author:
Christian Theobalt is a Professor of Compter Science and the head of the research group "Graphics, Vision, & Video" at the Max Planck Institute for Informatics. From 2007 until 2009 he was a Visiting Assistant Professor in the Department of Computer Science at Stanford University. He received his MSc degree in Artificial Intelligence from the University of Edinburgh, his Diplom (MS) degree in Computer Science from Saarland University, and his PhD in Computer Science from MPI Informatik.
Most of his research deals with algorithmic problems that lie on the boundary between the fields of Computer Vision and Computer Graphics, such as dynamic 3D scene reconstruction and marker-less motion capture, computer animation, appearance and reflectance modeling, machine learning for graphics and vision, new sensors for 3D acquisition, advanced video processing, as well as image- and physically-based rendering.
For his work, he received several awards including the Otto Hahn Medal of the Max Planck Society in 2007, the EUROGRAPHICS Young Researcher Award in 2009, and the German Pattern Recognition Award 2012. He is also a Principal Investigator and a member of the Steering Committee of the Intel Visual Computing Institute in Saarbrücken.
contact: theobalt (at) mpi-inf.mpg.de
resources.mpi-inf.mpg.de/perfcap/