Clothing: Estimating 3D Humans in Clothing from Images and Video
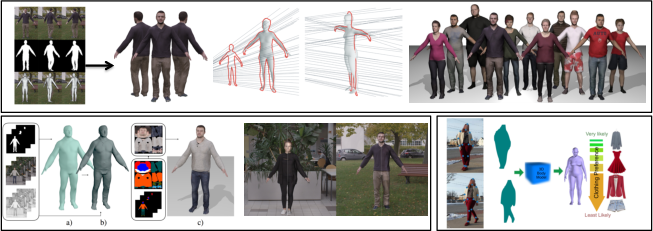
Clothing. Top: In [1] we can reconstruct human body shape, clothing and appearance from a single monocular video. The core of the method is a geometric optimization algorithm which brings pose varying silhouettes into a an un-posed reference frame. This allows to fuse the shape information of every frame. Bottom-right: We extended the approach to recover sharp textures and fine-detail [2]. Bottom-left: Using automatic visual shape inference we studied the correlation between body shape and clothing preference [3].
Understanding human behavior is not only about motion and body shape. The type of clothing people wear is another form of expression. People use clothing to express their political views, age, gender or social status. Instead of inferring body pose and shape while being invariant to clothing, we aim at perceiving and capturing human body shape along with clothing (category, appearance and shape) from images.
In [1] we describe a method to obtain accurate 3D body models alongside clothing and texture of arbitrary people from a single, monocular video in which a person is moving–we achieve a reconstruction accuracy of 4.5mm. This is the first method capable of reconstructing people and their clothing from a single RGB video without using a pre-scanned templates. At the core of our approach is the transformation of dynamic body pose into a canonical frame of reference. Our main contribution is a method to transform the silhouette cones corresponding to dynamic human silhouettes to obtain a visual hull in a common reference frame. This enables efficient estimation of a consensus 3D shape, texture and implanted animation skeleton based on a large number of frames. Results on 4 different datasets demonstrate the effectiveness of our approach to produce accurate 3D models, see Figure-Top. Requiring only an RGB camera, our method enables everyone to create their own fully animatable digital double, e.g., for social VR applications or virtual try-on for online fashion shopping.
We further extended the approach in [2] by integrating shading cues and a graph based optimization for body texture generation from a single RGB-video, see Figure-Bottom. While accurate, these approaches [1,2] are based on non-linear optimization, which can fail when they are not initialized correctly, and are typically slow. Hence, in recent work [4] we propose an approach that combines the benefits of deep learning and model based fitting together. The network takes multiple video frames as input and produces bottom-up a single coherent shape and a 3D pose for each of the frames. The estimates are optimized top-down in order to maximize the silhouette overlap and minimize the re-projection error. This results in accurate predictions in less than 10 seconds.
Understanding people clothing preferences according to body shape is another important problem with lots of practical applications–the most prominent one perhaps is for effective clothing recommendation in order to minimize returns. Our key idea is to leverage the large photo-collections in the internet to study the correlation between body shape and clothing preference [3]. To that end, we propose a novel inference technique capable of estimating body shape under clothing given a few images of the same person in different clothing. With these shape estimates and the predicted clothing categories from the images, we compute a distribution of clothing category conditioned on body shape. Using our method, we found out that clothing is indeed correlated with body shape, and we can predict clothing categories based on automatic shape estimation.
References
[1] Video Based Reconstruction of 3D People Models
T. Alldieck, M. A. Magnor, W. Xu, C. Theobalt and G. Pons-Moll
IEEE/CVF Conference on Computer Vision and Pattern Recognition (CVPR 2018)
[2] Detailed Human Avatars from Monocular Video
T. Alldieck, M. A. Magnor, W. Xu, C. Theobalt and G. Pons-Moll
3DV 2018 , International Conference on 3D Vision, 2018
[3] Fashion is Taking Shape: Understanding Clothing Preference Based on Body Shape From Online Sources
H. Sattar, G. Pons-Moll and M. Fritz
2018 IEEE Winter Conference on Applications of Computer Vision (WACV 2019), 2019
[4] Learning to Reconstruct People in Clothing from a Single RGB Camera
T. Alldieck, M. A. Magnor, B. L. Bhatnagar, C. Theobalt and G. Pons-Moll
32nd IEEE Conference on Computer Vision and Pattern Recognition (CVPR 2019), 2019