Classifier Based Graph Construction for Video Segmentation
Anna Khoreva, Fabio Galasso,
Matthias Hein and Bernt Schiele
Abstract
Video segmentation has become an important and active research area with a large diversity of proposed approaches. Graph-based methods, enabling top-performance on recent benchmarks, consist of three essential components: 1. powerful features account for object appearance and motion similarities; 2. spatio-temporal neighborhoods of pixels or superpixels (the graph edges) are
modeled using a combination of those features; 3. video segmentation is formulated as a graph partitioning problem. While a wide variety of features have been explored and various graph partition algorithms have been proposed, there is surprisingly little research on how to construct a graph to obtain the best video segmentation performance. We propose to combine features by means of a classifier, use calibrated classifier outputs as edge weights and define the graph topology by edge selection. By learning the graph (without changes to the graph partitioning method), we improve the results of the best performing video segmentation algorithm by 6% on the challenging VSB100 benchmark, while reducing its runtime by 55%, as the learnt graph is much sparser.
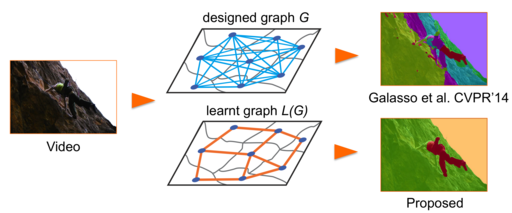
Results
Code and Data
References
[Khoreva et al., 2015] Classifier Based Graph Construction for Video Segmentation, A. Khoreva, F. Galasso, M. Hein and B. Schiele, Computer Vision and Pattern Recognition (CVPR), June, (2015)
@inproceedings{khoreva15cvpr,
title={Classifier Based Graph Construction for Video Segmentation},
author={A. Khoreva and F. Galasso and M. Hein and B. Schiele},
booktitle={Proceedings of the IEEE Conference on Computer Vision and Pattern Recognition (CVPR)},
year={2015}}