People Detection and Tracking
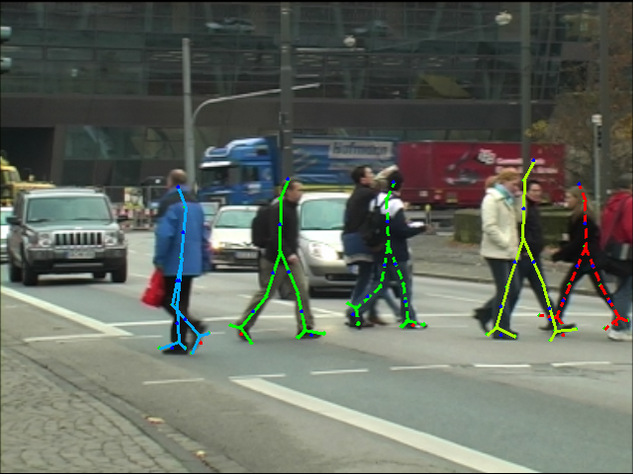
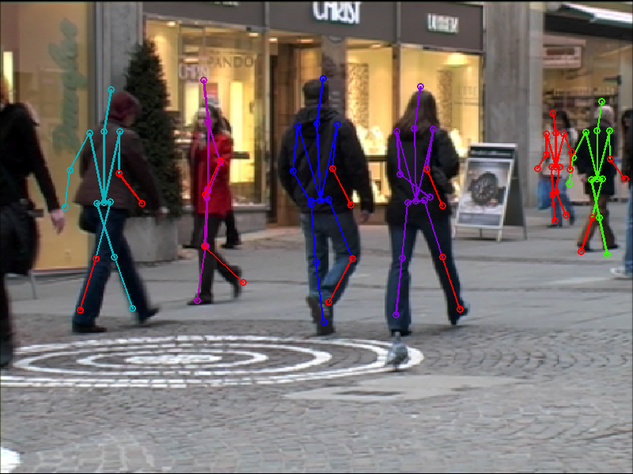
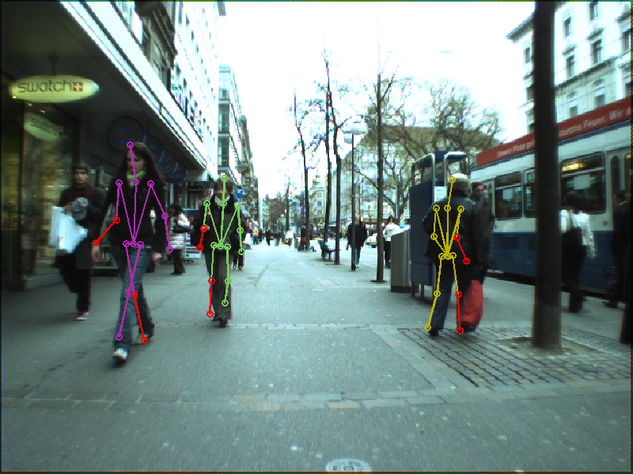
Finding and following people is a key technology for many applications such as robotics and automative safety, human-computer interaction scenarios, or for indexing images and videos from the web or surveillance cameras. At the same time it is one of the most challenging problems in computer vision and remains a scientific challenge for realistic and challenging scenes.
We developed a new approach for detecting people and estimating their poses in complex street scenes with multiple people and dynamic backgrounds. Our approach does not require multiple synchronized video streams and can operate on images from a moving, monocular and uncalibrated camera. The important challenges addressed in our approach are frequent full and partial occlusions of people, cluttered and dynamically changing backgrounds, and ambiguities in recovering 3D body poses from monocular data.
Several key components contribute to the success of the approach. The first is a novel and generic procedure for people detection and 2D pose estimation [1] that is based on the pictorial structures model and also enables to estimate viewpoints of people from monocular and single images.
Our approach [1] is based on the discriminatively learned local appearance models of the human body parts that are combined with a flexible kinematic-tree prior on part configurations. The appearance of the body parts is represented by a set of densely computed shape context image descriptors, which correspond to local distributions of gradient orientations captured for the points on the image edges. We employ a boosting classifier trained on the dataset of annotated human poses in order to learn which of these local features are informative for the presence of the body part at a given image location. Interpreting the output of each classifier as a local likelihood we infer the optimal configuration of the body parts using belief propagation.
The second key component of our approach enables people tracking and monocular 3D pose estimation [2,3]. In contrast to prior work we accumulate evidence in multiple stages thereby reducing the ambiguity of 3D pose estimation effectively in every step.
In [2] we propose a novel multi-stage inference procedure for 3D pose estimation. Our approach goes beyond prior work in this area, in which pose likelihoods are often based on simple image features such as silhouettes and edge maps and which often assume conditional independence of evidence in adjacent frames of the sequence. In contrast to these approaches, our 3D pose likelihood is formulated in terms of estimates of the 2D body configurations and viewpoints obtained with a strong discriminative appearance model. In addition we refine and improve these estimates by tracking them over time, which allows to detect occlusion events and group hypotheses corresponding to the same person. We demonstrate that the combination of these estimates significantly constrains 3D pose estimation and allows to prune many of the local minima that otherwise hinder successful optimization.
References
[1] Pictorial Structures Revisited: People Detection and Articulated Pose Estimation, M. Andriluka, S. Roth and B. Schiele, IEEE Conference on Computer Vision and Pattern Recognition (CVPR), (2009)
[2] People-Tracking-by-Detection and People-Detection-by-Tracking, M. Andriluka, S. Roth and B. Schiele, IEEE Conference on Computer Vision and Pattern Recognition (CVPR'08), (2008)
[3] Monocular 3D Pose Estimation and Tracking by Detection, M. Andriluka, S. Roth and B. Schiele, IEEE Conference on Computer Vision and Pattern Recognition (CVPR), 06/2010, San Francisco, USA, (2010)